Elevating Enterprise BI: Advanced
Techniques for Maximizing ROI and
Driving Innovation in Mature Data Environments
Organizations with mature BI systems face the challenge of pushing beyond existing boundaries to extract more value, drive innovation, and automate decision-making. The goal is to enhance BI capabilities and ensure the organization stays ahead in an increasingly competitive business landscape.
Talk to us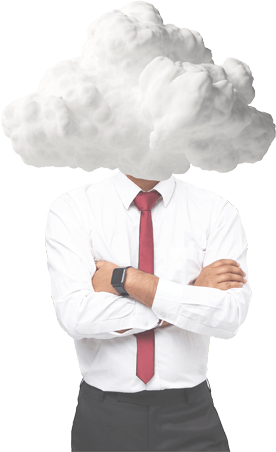
Advanced data analysts now focus on maximizing ROI by enhancing BI efficiency, extracting deeper insights, and delivering greater strategic impact to elevate their businesses. As data leaders, your role extends beyond managing the BI infrastructure. It's about discovering new opportunities for growth by leveraging advanced tools, predictive models, and optimization techniques.
We'll delve into advanced concepts that go beyond the basics, assuming a strong foundation in BI principles and practices.
1. Leveraging AI and Machine Learning in BIThe integration of Artificial Intelligence (AI) and Machine Learning (ML) into BI systems represents one of the most significant opportunities for advancement in mature data environments. While predictive analytics has been around for some time, the key now lies in seamlessly embedding these capabilities into everyday BI workflows.
Advanced implementations are moving towards real-time predictive analytics integrated directly into dashboards. This allows decision-makers to not only see what has happened but also what is likely to happen next, all within the same interface. For instance, a sales dashboard could include AI-driven forecasts that automatically update based on the latest data, providing instant insights into future performance.
Natural Language Processing (NLP) is another frontier in AI-enhanced BI. Conversational BI interfaces allow users to interact with data using natural language queries, dramatically lowering the barrier to data access across the organization. Imagine executives being able to ask complex questions about business performance and receiving instant, AI-generated insights without needing to navigate complex dashboards or write queries.
Automated anomaly detection and alerting systems, powered by machine learning algorithms, are becoming increasingly sophisticated. These systems can learn from historical data patterns to identify subtle anomalies that human analysts might miss, alerting relevant stakeholders in real-time to potential issues or opportunities.
2. Advanced Data Governance in Complex EcosystemsAs BI environments mature and expand, maintaining data quality and governance becomes increasingly challenging. Advanced data governance strategies are essential for ensuring the reliability and trustworthiness of insights derived from BI systems.
Implementing federated governance models can help balance the need for centralized control with the flexibility required by different business units. This approach allows for standardized data definitions and quality controls while enabling departments to manage their data assets in ways that best suit their specific needs.
In multi-cloud environments, balancing security and accessibility is a complex but critical task. Advanced techniques involve implementing granular access controls, encryption, and auditing mechanisms that work seamlessly across different cloud platforms. Zero-trust security models, combined with AI-driven threat detection, can provide robust protection without impeding legitimate data access and analysis.
3. Real-time Analytics OptimizationFor many organizations, the ability to process and analyze data in real-time is becoming a critical competitive advantage. However, achieving true real-time analytics at scale presents significant technical challenges.
Advanced techniques for reducing latency in large-scale data processing include the use of in-memory computing, optimized data storage formats like Apache Parquet, and sophisticated caching strategies. Stream processing frameworks such as Apache Flink or Kafka Streams can be leveraged to build highly efficient real-time analytics pipelines.
Edge analytics is becoming increasingly important, especially for organizations dealing with IoT data. By processing data at the edge, close to where it's generated, organizations can reduce latency, lower bandwidth costs, and enable faster decision-making. Implementing effective edge analytics requires careful consideration of hardware limitations, network connectivity, and data synchronization strategies.
4. Advanced Data Visualization TechniquesWhile basic charts and graphs remain important, advanced BI environments are pushing the boundaries of data visualization to enable deeper insights and more intuitive data exploration.
Interactive, drill-down capable dashboards allow users to seamlessly move from high-level overviews to granular details. Techniques like guided analytics can provide users with AI-assisted exploration paths, helping them uncover insights they might otherwise miss.
Augmented Reality (AR) for data visualization is an emerging field with exciting potential. AR can overlay data visualizations onto the physical world, providing context-rich insights. For example, a manufacturing plant manager could use AR glasses to view real-time performance metrics overlaid on actual machinery.
When dealing with complex, multi-dimensional data, traditional visualization techniques often fall short. Advanced techniques like parallel coordinates plots, Sankey diagrams, or force-directed graphs can help users understand complex relationships and patterns in high-dimensional data sets.
5. Cross-functional Data IntegrationBreaking down data silos and achieving a truly integrated view of the business remains a challenge even for mature BI environments. Advanced approaches to this problem go beyond simple data warehousing.
Data mesh architectures represent a paradigm shift in how organizations think about data integration. Instead of centralizing all data, a data mesh treats data as a product, with each domain responsible for providing high-quality, easily accessible data to the rest of the organization. This approach can lead to more agile, scalable data ecosystems.
Techniques for harmonizing disparate data sources have also evolved. Advanced semantic layers can provide a unified view of data across different systems, even when the underlying data models differ significantly. Graph databases are increasingly being used to model and analyze complex relationships across diverse data sets.
6. Ethical Considerations in Advanced AnalyticsAs BI systems become more powerful and pervasive, ethical considerations become increasingly important. Advanced practitioners must grapple with issues of bias, privacy, and transparency.
Addressing bias in AI-driven BI systems is critical. This involves not only careful data preparation and model training but also ongoing monitoring and adjustment. Techniques like adversarial debiasing or multi-objective optimization can help create fairer, more equitable AI models.
Privacy-preserving analytics techniques, such as differential privacy or homomorphic encryption, allow organizations to derive insights from sensitive data without compromising individual privacy. These techniques are becoming increasingly important in light of stringent data protection regulations.
Ensuring transparency and explainability in complex models is another key challenge. Techniques like SHAP (SHapley Additive exPlanations) values or LIME (Local Interpretable Model-agnostic Explanations) can help make black-box AI models more interpretable, which is crucial for building trust and meeting regulatory requirements.
7. Performance Tuning for Large-scale BI EnvironmentsAs BI systems grow in scale and complexity, performance tuning becomes increasingly critical. Advanced query optimization techniques go beyond basic indexing and partitioning strategies.
Techniques like query rewriting, where complex queries are automatically restructured for optimal performance, can significantly improve response times. Cardinality estimation, crucial for query optimization, is being enhanced with machine learning techniques to provide more accurate estimates, leading to better query execution plans.
Intelligent caching strategies, which predict which data is likely to be needed and pre-cache it, can dramatically improve performance for frequently accessed data. This can involve sophisticated algorithms that learn from usage patterns and adapt in real-time.
GPU acceleration for analytics is becoming more mainstream, offering orders of magnitude improvements in performance for certain types of computations. Leveraging GPUs effectively requires careful consideration of data transfer overhead and algorithm design.
8. Measuring and Maximizing BI ROIIn mature BI environments, demonstrating and maximizing ROI becomes more nuanced. Advanced KPIs for measuring BI effectiveness go beyond simple usage metrics to assess the actual business impact of BI initiatives.
Value stream mapping, a lean management technique, can be applied to BI processes to identify and eliminate waste, streamline workflows, and maximize value delivery. This approach can help organizations optimize their BI investments and ensure they're focusing on high-impact activities.
Quantifying indirect benefits of BI initiatives, such as improved decision-making quality or increased innovation, remains challenging but is crucial for understanding the full value of BI investments. Advanced techniques might involve sophisticated modeling of decision processes or longitudinal studies of organizational performance.
9. Future-proofing BI InfrastructureEnsuring that BI infrastructure can adapt to future needs is critical in fast-evolving technology landscapes. Strategies for scalable and flexible BI architectures often involve modular designs that can easily incorporate new technologies or scale to meet growing demands.
Implementing containerization and microservices in BI allows for more flexible, scalable, and resilient systems. This approach enables organizations to more easily update or replace individual components of their BI stack without disrupting the entire system.
While still in its early stages, quantum computing has the potential to revolutionize certain aspects of data analytics, particularly in areas like optimization and simulation. Forward-thinking organizations are already exploring how quantum computing might be integrated into their BI and analytics workflows in the future.
ConclusionAs we've explored, elevating enterprise BI in mature data environments involves pushing the boundaries across multiple fronts – from advanced AI integration and real-time analytics optimization to ethical considerations and future-proofing infrastructure. For advanced data analysts and BI professionals, the key lies in continuously exploring new techniques and technologies while always keeping sight of the ultimate goal: delivering actionable insights that drive business value.
The future of BI is not just about technology, but about how we can leverage these advanced capabilities to foster a truly data-driven culture within organizations. By focusing on these cutting-edge areas, BI professionals can ensure that their mature data environments continue to evolve, innovate, and deliver ever-increasing value to their organizations.
-
September 06, 2024
Join our the insight community and get exclusive updates, industry news, and practical resources to drive your business forward.